Biostatistics and Biometrics - Juniper Publishers
Abstract
Traditional parallel group double-blind controlled trial design is the preferred approach to provide substantial evidence to support a new drug application. To elevate trial efficiency to a new level, the pharmaceutical industry and the health regulatory authorities continuously make great effort to propose the applications of innovative designs to new drug developments. These designs include the branching out group sequential design, a variety of adaptive designs and platform designs. Moreover, to overcome the challenge of patient recruitment and reduce trial sample size particularly in rare disease areas, Bayesian design and patient matching approach are often applied by incorporating historical or real-world data for statistical inference. In addition, for ethical or practical considerations, single arm or open label randomized controlled designs may also be utilized to evaluate treatment effects. In this paper, we discuss these designs and their applicability in different scenarios, in the context of proposing a new pseudo randomized controlled design where patients ‘randomized’ to control will be under standard of care and therefore may not need to consent for the interventional trial procedures and need only agreeing the use of their data which are closer to real-world data. This new design improves upon the single arm design, while featuring part of the open label randomized controlled designs; thus, inherit some of their key features as we summarize in the paper. Therefore, this new design may have its value in some trial settings through providing improved controlled Real-World Data..
Keywords: Bayesian Design; Historical Data Borrowing; Single Arm Design; Open Label Randomized Design; Real-World Evidence; Pseudo Randomized Controlled Design
Introduction
Currently, with the cost of developing a new medicine continuously increases, paradigm for clinical research has changed over time. New ideas for a variety of innovative clinical trial designs steadily emerge. Regulatory agencies also start to accept some of these designs [1] for the purpose of accelerating the availabilities of more innovative, safe and effective therapies to patients. Nonetheless, we should still open our mind to think of and embrace all other possible clinical trial designs. In this paper, we will discuss the key features of some these designs, motivating us to propose a new design: pseudo randomized controlled design, which could be considered as a design improving upon the traditional single arm design and open label randomized controlled design. The new design can also leverage Real-World Data while address selection biases and comparability concerns. With the layout of all the design options, it will be convenient for the practitioners to compare and evaluate the choices for a specific clinical study.
Randomized Double-Blind Controlled Trial Designs
The traditional randomized double-blind controlled trial design is considered as the ‘gold standard’ design. In this design, the sponsor first prepares a study protocol which details the objectives/hypotheses, the sample size, the study population (inclusion/exclusion criteria), the study duration, the number of visits, the treatments which include the control, the methods of endpoint measurements, the other study procedures and the approaches for data analysis (e.g., the model, the estimand and the way for handling missing data). Then the sponsor selects study centers from different countries based on the feasibility of timely recruiting patients and the regional sample size requirements from the regional health authorities [2-4]. Often after a run-in period, patients are randomized to treatment or control after consent and go through the study procedures specified in the protocol. Trial results are subsequently derived after data base lock. The common perception is that trial results with such a design are ‘unbiased’ for ‘certain measures, estimands and purposes.
Actually, trial results even from a trial with such a ‘gold standard’ design are still trial specific with the limitation for the particular population via the country or site selections and protocol inclusion/exclusion criteria. They are unbiased only within this population as well as the study procedures described in the protocol including perhaps the inclusion of the run-in qualification period. To have the evidence of a robust (beyond this narrow study population) treatment effect, the sponsors are often required to conduct two pivotal studies (or a large single trial with an extremely small p-value) at different study centers/countries. Both studies should confirm the treatment effect to demonstrate the reproducibility. Additional evidence could be provided through trials for additional related indications. Moreover, post-marketing bridging studies may be conducted in new countries to extrapolate the treatment effect to different/broader patient populations. Real-world or post-marketing observational studies may also be conducted to get real-world evidence in a real-world setting. Even though study centers are not randomly selected globally, results derived from a random effect model treating study center as a random effect with the incorporation of between-center variability should be more generalizable than those from a fixed effect model [5-7]. Nonetheless, we seldom see the application of the random effect model to multi-center trials or multi-regional trials. Subgroup analyses need also being performed to assess the consistency of treatment effects across different subpopulations.
Within the broad traditional randomized controlled design category, there are further subtypes of designs. They include the fixed design, group sequential design, sequential parallel comparison design, platform design, adaptive design, the regular parallel group design and crossover design. Different designs have their own advantages under their corresponding assumptions and circumstances. For example, owing to many unknowns before the initiation of a study, we have the desire to modify the study based on either the blinded or unblinded internal data of the study obtained during the trial monitoring process to increase the chance of trial success. We then can apply the adaptive design which provides us many flexibilities [8]. We can stop the trial early due to futility or unexpectedly strong treatment effect. We can revise the sample size for the targeted conditional power. We can select dose(s) and enrich patient population(s) for the later stages of the trial. We can even determine for the final analysis whether we want to only assess the non-inferiority or go further to assess the superiority if there is the hope based on the interim data. The power for superiority assessment will be no larger than the significance level if there is truly no between-treatment difference regardless of the magnitude of the sample size. If the interim data show no or very small treatment effect, we had better just go for the noninferiority assessment so that we can complete the study early and save valuable resource [9].
Given the limitation of the trial results from the traditional randomized controlled trials revealed above, we are motivated to explore other possible alternative trial designs. One ideal scenario is to find all patients without applying the extensive inclusion/exclusion criteria and randomize them to treatment and control. Even though the trial results (the focus should be on estimation rather hypothesis testing with the huge sample size) are the results of one specific realization of the randomization and another similar trial cannot be conducted after (almost) all patients have been studied (unless another trial is conducted in newly diagnosed patients subsequent to the first trial), the trial results should be applicable to another randomization of the same scheme due to the huge scale of the trial. Thus, the results should be more generalizable compared to those of the traditional randomized controlled trials with limited sample sizes. Clearly, such a design is not realistic as we cannot really include all (currently diagnosed) patients in one single study. In any case, we should keep in mind that trial results from a larger trial are always more desirable and interpretable than those of a relatively smaller trial [10]. The size of a trial should be determined not merely for enough power for hypothesis testing of the primary efficacy endpoint but more for appropriate and robust quantitative evaluation of the treatment effects measured broadly by other endpoints including those for safety assessments. As discussed below, other considerations can trigger the applications of alternative designs given the infeasibility of the various traditional randomized controlled trial in some circumstances.
Single Arm Design
It is sometimes unethical or practical to include a (placebo) control in a clinical study particularly exposing a large number of patients to ineffective/inappropriate placebo control. In addition, patients may not have the motivation to participate in such placebo-controlled trial if alternative treatments are available in the disease area which makes recruitment very challenging. Then a single arm design will often be the option for the study. EMA recently issued a draft reflection paper on establishing efficacy based on single-arm trials [11]. The reflection paper clearly outlines the description and specific characteristics of single-arm trials. It also provides general considerations for single-arm trial designs including the choice of endpoints, target and trial population, role of external information, statistical principles, sources of bias and potential mitigation. As there is no internal control, the endpoints must be able to isolate treatment effects undoubtedly caused by the treatment rather than the selection of trial population or some prognostic factors. The assessment of treatment effect may be through the evaluation of the within-treatment effect; for example, measured by mean change from baseline at a specific time point for a continuous endpoint and compared to a threshold.
This threshold often depends on the treatment effect of the standard of care (SOC) (measured using historical data). Notice that the treatment effect of the standard of care can improve or change over time along with the continuous advancement of innovative medical technology and the availability of more effective treatments or other prior or concomitant medical interventions. It may also depend on the population and the regions of conducting the study as the standard of care can vary substantially long with the health care system and even the human development index [12] across countries. In addition, if the threshold is a constant without any variability, the study should be sized with certain level of precision based on confidence interval for the assessment of treatment effect. Clearly, the determination of a relevant threshold is not straightforward, and the interpretation of the trial result may rely on something that is not measured within the study. Such studies therefore are more limited to rare diseases and oncological studies [13], where the effect of ‘current standard of care’ is usually quite low with small variabilities. For binary, count data, time to event and other study endpoints, the within-treatment event rate, cumulative event rate curve and the other summary statistics may be used to evaluate the within-treatment effect.
Rather than comparing the within-treatment effect to a constant threshold, we may directly leverage external historical control data or Real-World Data (RWD) (potentially concurrent standard of care control) using a synthetic control for statistical inference of a single arm trial in order to consider the data variability (rather than just the use of the point estimate as the threshold). But the results will still be uninterpretable if we fail to account for known and unknown confounding factors when comparing the results to an external control [13]. Thus, when external data are borrowed to form a control group, for the validity of the analysis outcome, we need to ascertain some key assumptions which include the consistency of patient population, medical practices, and treatment regimens. If individual patient data from the external source are available, propensity score estimated from individual patients’ covariates can be applied to match and select patients from the external source [14]. Thus, patients of the single arm trial and the selected patients from the external source are comparable at least by some measures believed to be relevant or predictive of ‘disease progression’. If individual patient data from multiple RWD sources are not simultaneously accessible due to data privacy policy, aggregated data in the form of special summary statistics may be requested in order to perform the appropriate data matching analysis [15,16]. Further research in this area is of great critical needs.
As for the other open label studies, to mitigate potential bias, for a single-arm trial, the statistical analysis plan should be finalized before the initiation of the study. Theoretically, during the trial, there should be no unplanned trial modifications including the target population, the sample size, the threshold, the approach for analysis and the source of external information. Any amendment is considered potentially data driven.
Historical Data Borrowing Design and Pseudo Randomized Controlled Design
Obviously, there are some challenging issues associated with the traditional single arm design in the alternative design category as alluded to previously. We attempt here to address the issue of better generating and incorporating valid internal/external control data to ameliorate the design deficiency of single arm studies.
Bayesian Design
Bayesian design allows the leveraging of historical/external information through the application of an informative prior distribution. The design may borrow treatment effect information (both the active treatment and control data) or borrow just the control data from the historical studies so that the total sample size of the current study can be reduced to make the study more feasible particularly for a trial in a rare disease area where recruiting patients is very challenging. If only the historical control data will be borrowed, a full sample size for the experimental treatment arm, but a reduced sample size for the control arm could be used. Different from the single arm trials, the study with a Bayesian design has both comparative arms even though the sample size for the control arm may be relatively smaller. Thus, the design can sometimes be treated as the hybrid of single arm and controlled design with the borrowing of external data. Only when internal data are available can we assess the consistency between the historical and current data. Consistency has two aspects. One is the consistency in study setting regarding patient population definition/identification, study endpoint definition/specification, study procedures and similar study centers [17].
This consistency is the prerequisite for the validity of the design. Another aspect is the consistency in the study endpoint value across the historical and current studies. To prevent the excessive amount of data borrowing, even when the study characteristics of the historical and current studies are largely similar, a dynamic data borrowing procedure may still be implemented so that the amount of data borrowing depends on the observed level of consistency in endpoint value (e.g., the observed mean values of the endpoint) of the two datasets [18,19]. The amount of data borrowing is reflected in the adjustment on the variance of the prior distribution or the effective sample size. There is no impact on the mean of the prior distribution in this dynamic historical data borrowing process. If baseline covariates could impact the value of the study endpoint, to make the patients of the historical data comparable to the concurrent patients, we can first do patient matching and selection using propensity score and then apply the Bayesian dynamic approach to regulate the amount of data borrowing.
A Bayesian design is considered as a Complex Innovative Design (CID) per the FDA guidance [6]. CIDs are designs that have rarely or never been used to date to provide substantial evidence of effectiveness in new drug applications or biological license applications. Based on the guidance, extensive simulation should be performed to demonstrate the frequentist operating characteristics of the Bayesian design in terms of type I error probability and power. Rather than just claiming the significance of the treatment effect and providing the point estimate of the treatment effect, the advantage of the Bayesian design and analysis is that it provides the flexibility to make probabilistic statement regarding the true treatment effect through the posterior distribution. Particular attention should be paid to any historical borrowing methods. With the improvement of the standard of care, placebo effect may vary and change over time, and the treatment effect may attenuate over time. Therefore, the borrowing of historical control data may create bias in favor of the superiority claim but against the non-inferiority claim [20]. Simulation will be helpful to quantify the range of bias.
Pseudo Randomized Controlled Design
One of the underlying deficiencies with the above
single arm or Bayesian design is the usage of ‘historical’ control data
which are subject to drift over time, and therefore potentially
non-comparable to current medical practices, endpoints measurements,
study procedures and patient populations. The question is whether we can
and how to potentially address this challenge (at least in part). Let’s
now modify the traditional randomized controlled open label trial in
the following way. In selected countries/sites, patients who meet the
protocol criteria will be virtually ‘randomized’ to the treatment or
control in a pseudo trial. There is no need for the patients to know
they are randomized in this ‘pseudo’ trial at this point of time. Those
who are ‘randomized’ to the control will be followed with the standard
clinical practice procedure or standard of care, in this case, without
the need of consent. By nature of this randomization scheme, this
‘control’ group is (concurrent and) comparable to the future ‘treatment’
group and serves as the basis of creating ‘unbiased’ comparisons. Among
the patients who are ‘randomized’ to the treatment, we ask for their
informed consent to participate in an open label single arm trial. Those
who consent to participate in the pseudo randomized and ‘single
treatment arm’ study will take the study medication and be followed
according to the protocol while the other patients who do not consent
will be followed like those ‘randomized’ to the control, i.e., under the
standard of care. (Figure 1) provides the flow chart of this design
which has the following features.
i.It can be considered as a design with a single treatment arm plus a
‘randomized’ concurrent rather than historical control arm. Therefore,
this design is somewhere between the single arm uncontrolled or
historical controlled design and the regular open label randomized
controlled design. Note that the traditional single arm trial does not
have a randomization step and therefore has no randomized ‘unbiased’
concurrent control. The issues associated with the traditional single
arm trials have been discussed in Section 3. This design improves upon
the traditional single arm trial by providing a comparable concurrent
control group and yielding ‘unbiased’ treatment effect estimates.
ii.Note further that patients are ‘randomized’ first and then consent
next for those randomized to ‘treatment’, or consent to provide Real
World data for those randomized to ‘control/placebo’. In the traditional
randomized controlled trials, patients consent first then are
randomized later, those who do not consent are not randomized and not
included in either the treatment arm or control arm. The proposed new
design could therefore be applicable to situations where traditional
randomized, placebo-controlled trials are not ethical or practical.
iii.The control is the real open label standard of care control rather
than a placebo control (with the exclusion of certain concomitant
medications). Due to the open label nature, if a placebo was used, there
should be no extra placebo effect (Figure 1).
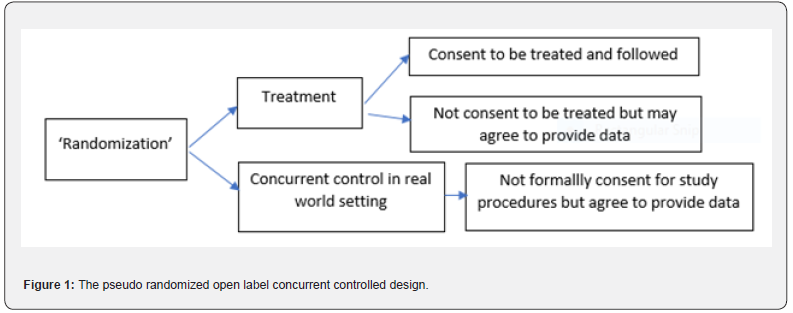
We next address the question of how to perform data analysis and interpret the results; moreover, how to use the data from patients who are randomized to ‘treatment’ but do not consent. First, for those patients randomized to treatment, we need to assess the comparability between those who consent and do not consent to participate in the study based on their characteristics (In traditional trials, we cannot assess this. It is nice to have this information). In the case where they are very similar, we can infer that if the patients who are randomized to the control were also asked to consent, likely, those who consent and those who do not consent might also be similar. Actually, no matter whether they consent or not, they all should realize that they will all have the same standard of care. Thus, the comparison between the treated patients and all the patients in the control arm should be justifiable. Patients who are randomized to treatment, but do not consent have no on-treatment data. If the intent-to-treat principle is applied and include all randomized patients in the analysis, clearly, the treatment effect will be substantially underestimated. As the missing data mechanism is more likely to be independent with the missing endpoint data and therefore is missing at random. The analysis based on a likelihood approach with non-consent patients excluded is therefore valid [21,22] particularly if the key baseline covariates are included in the analysis model. On the other hand, in case where the consent and none consent patients are different in terms of their characteristics, propensity score can be used for patient matching and selection from the controlled patients for the eventual treatment effect evaluation [14]. Since the control is concurrent real-world control and ‘randomization’ is performed, the between treatment comparison should be less biased and we should have more confidence with this analysis compared to the analysis with historical control.
Note that in order to include patients in data
analysis, we need to obtain their agreement or ‘consent’ to use their
data. This ‘consent’ is a light consent (e.g., signing a HIPPA release
form with appropriate de-identification measures) for those who are
randomized to the control as they do not need going through
interventional study procedures. We just need their endpoint being
measured at specific timepoints, which can be considered as a part of
the treatment procedures even under the standard of care. Since the
safety profile of the standard of care should be well known, patients in
the ‘control’ group also do not need to take the cumbersome safety
endpoint measurements. The safety of the experimental drug can be
evaluated by the within treatment assessment approach using the
concurrent background safety profile as the reference. Moreover:
i.For efficacy: we likely only have measures of the primary endpoint
(survival or clinical outcome) at certain timepoints. Study design
should consider such SOC practices, so we avoid any design or data
measurement bias (e.g, more frequent measures in treatment group,
creating ascertainment biases). For some secondary endpoints, we may not
have data from the control group.
ii.For safety, again, we need to avoid data collection biases: if we
have more frequent clinical visits in the treatment group, we may
collect more ‘routine’ adverse events or have more lab abnormalities
detected via frequent central labs. Therefore, we should focus more on
serious adverse events, or assess lab out of ranges (exceeding multiple
times of the upper limits of normal) only at timepoints of SOC practice.
If all patients are required to formally consent and go through the formal study procedures according to the trial protocol, the pseudo randomized design can be replaced by a traditional randomized open label-controlled design. (Table 1) provides the summary of the comparative features of different designs. Besides those discussed in (Table 1), for the pseudo randomized trial design, trial procedures could be simplified at least for the patients in the control group to save cost. Moreover, there will be less issues of patients’ dropping out from the ‘study’ for those randomized to the control (Table 1).
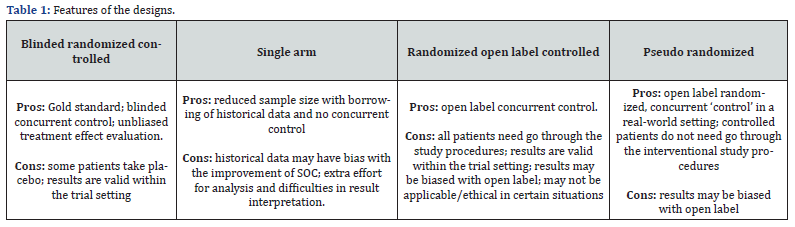
Discussion
As discussed, there are many different clinical trial designs for us to select for a specific trial in a given context. The suitability of a design will depend on the disease area, the objectives of the trial, the nature of the new treatment, the study population, the acceptability by the health authorities, the timeline and cost considerations. Some of the designs including the traditional randomized double-blind controlled design and single arm design are widely used. Nonetheless, they may not always be applicable efficient or feasible for some scenarios. Thus, innovative designs are proposed in literature and are being used in practice. Clearly, some of these designs may need additional assumptions for the results to be valid such as the consistency assumption for the use of the Bayesian design with historical data borrowing. Some of the assumptions can be verified via observed data when performing data analysis. Based on the comparison in (Table 1), the pseudo randomized controlled design may be a suitable option when blinding is not possible, and we need a concurrent control to provide valid comparable data in a real-world setting where patients can have more flexibility and at the same time cost for the study can be reduced. There is one drawback compared to traditional single arm trials: because of the ‘pseudo randomization,’ we do ‘lose’ some patients who refuse to consent to be treated.
Even though there may be some sound rationale and considerations behind any new designs, to fully understand and appreciate the features of the design, we need to further evaluate and share our experience obtained from real trial practice. Sometimes, performing simulation for a specific trial setting is also necessary per the regulatory guidance. In terms of the pseudo randomized single treatment arm concurrent controlled design discussed in Section 4.2, we do not have much experience regarding when and why a patient would consent or not consent to participate in the active treatment group of the study. Patients’ behavior may depend on the availability of alternative medicines in the market even though a patient can always drop out from the study and switch medication any time during the study if the study treatment apparently has no effect. In addition, a patient may consent to take the active treatment and participate in the study for the purpose of saving medical cost and getting the needed care at the same time. Then the behavior will be ‘social-economic’ related and the associated prognostic covariates will need being incorporated in the analysis model to reduce bias. If such a design can be deployed, we will have data to assess the differences (if any) between consent and none-consent patient subgroups and evaluate the differences between the concurrent control group and any historical control data (if available). We welcome readers to consider and further enhance this new design proposal.
To Know more about Biostatistics and Biometrics Open Access Journal
Click here: https://juniperpublishers.com/bboaj/index.php
To Know more about our Juniper Publishers
Click here: https://juniperpublishers.com/index.php
No comments:
Post a Comment