Robotics & Automation Engineering - Juniper Publishers
Abstract
We would like to point out that reinforcement
learning (RL) needs to be used with caution in contingency planning for
UAVs. A more practical approach is proposed in this opinion.
Keywords: Contingency planning; UAVs; Reinforcement learning
Introduction
Reinforcement learning (RL) has two important
elements: “critic” and “reward” based on performance. As shown in Figure
1, the “reward” is generated based on a performance evaluator for a
system and the “critic” element generates proper actions to a system. In
recent years, RL has gained a lot of attention because of its success
in games such as the Alphago, which beat the human world champion in
straight sets [1]. However, there are several recent blogs [2-4] by
researchers in artificial intelligence (AI), who heavily criticized the
capability of RL in numerous applications. Some notable criticisms
include the requirement of huge amount of training data, lack of
mechanism to incorporate metadata (rules) into the learning process, the
requirement of starting from scratch in the learning process, etc. In
short, those researchers think that RL is not a mature technology yet
and has found success in only a few applications such as games,
collision avoidance, etc.
Drones, also known as Unmanned Air Vehicles (UAVs),
have much higher failure rates than manned aircraft [5]. In 2019, there
is a Small Business Innovative Research (SBIR) topic [6] seeking
ambitious ideas by using RL to handle quite a few contingencies in
drones, including 1) preset lost-link procedures; 2) contingency plans
in case of failure to reacquire lost links; 3) abort in case of
unexecutable commands or unavoidable obstacles; 4) terminal guidance.
After carefully analyzing the requirements in this topic, we believe
that a practical and minimal risk approach is to adopt a hybrid
approach, which incorporates both conventional and RL methods. Some of
the requirements in this topic can be easily handled by conventional
algorithms developed by our team. For instance, we have developed preset
lost-link procedures to deal with lost links for NASA. Our procedures
can satisfy FAA and air traffic control (ATC) rules and regulations. We
also have systematic procedures to deal with contingency in case of
failure to re-acquire lost links. All these procedures can be generated
using rules and do not require any RL methods. We believe that RL should
be best used in collision avoidance in dynamic environments be cause
there is mature development in using RL to tackle clutter
and dynamic environments for mobile robots.
Practical Approach
In the past seven years, our team has been working on contingency
planning for UAVs to deal with lost links for NASA and
contingency planning for engine failures, change of mission objectives,
missed approach, etc. for the US Navy. We also have a patent
on lost link contingency planning [7]. To tackle the contingencies
in drones, a practical approach has several components. First, we
propose to apply our previous developed system [7-10] to deal
with lost-link and other contingencies. Our system requires some
pre-generated databases containing FAA/ATC rules, locations of
communication towers, emergency landing places, etc. for a given
theater. Based on those databases, we can generate preset plans
to handle many of the contingencies related to lost-link, engine
failures, mission objective changes, etc. Our pre-generated contingency
plans can also handle terminal guidance. We plan to
devise different plans to handle different scenarios in the terminal
guidance process. For example, if the onboard camera sees a
wave-off signal, the UAV can immediately activate a contingency
plan to guide the UAV to an alternative landing place. Second, we
propose to apply RL to handle some unexpected situations such
as dynamic obstacles in the path. RL has been successfully used in
robot navigation in clutter and dynamic environments and hence
is most appropriate for collision avoidance in UAVs.
Figure 1 shows the relationship of our Advanced Automated
Mission Planning System (AAMPS), Joint Mission Planning System
(JMPS), and Common Control System (CCS). Our AAMPS first uses
JMPS to generate a primary flight plan for a given mission. JMPS
has the advantage of containing airport and constraint zone information
in its database. Second, we applied Common AAMPS
to generate contingency plans for the primary flight path. The
Ground Control System contingency plans can deal with major
situations such as engine out, lost communications, retasking,
missed approaches, etc. To avoid damage to ground structure, we
propose to apply an automatic landing place selection tool, which
selects appropriate landing places for UAVs so that UAVs can use
these landing sites during WEB Route normal flight or emergencies.
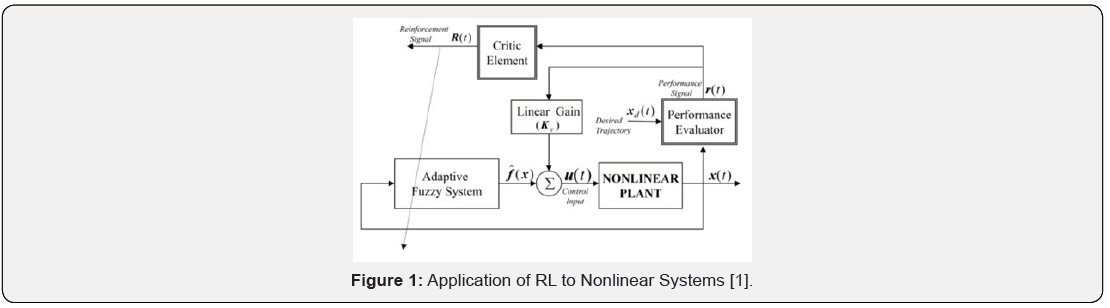
In our approach, RL is used for path planning to avoid dynamic
threats and obstacles. Recent works showed that RL could be
used for autonomous crowd-aware robot navigation in crowded
environments [11-12]. However, the performance of these techniques
degrade as the crowd size increases since these techniques
are based on a one-way human-robot interaction problem [13]. In
a recent paper [13], the authors introduces an interesting work
which uses RL for robot navigation in crowded environments. The
authors of [13] name their method Self- Attention Reinforcement
Learning (SARL). They also use the name local map SARL (LMSARL)
for the extended version of SARL. The authors approach
the crowd-aware navigation problem different than other techniques
and the human-human interactions which affects robot’s
anticipation capability for navigation are also considered in their
method, SARL [13]. SARL can anticipate crowd dynamics resulting
in time-efficient navigation paths and outperforms three state-ofthe-
art robot navigation in crowded scenes methods which are
Collision Avoidance with Deep Reinforcement Learning (CADRL)
[11], Long Short-Term Memory-RL (LSTM-RL) [12], and Optimal
Reciprocal Collision Avoidance (ORCA) [14]. We find similarities
between the crowd-aware robot navigation application and the
autonomous collision-free UAV navigation in crowded air traffic
and SARL can be used as a promising technique along this line. We
believe that we can customize SARL for autonomous collision-free
UAV navigation in contingency situations such as when the link
between operator and UAV is lost and the UAV needs to make a
forced emergency landing.
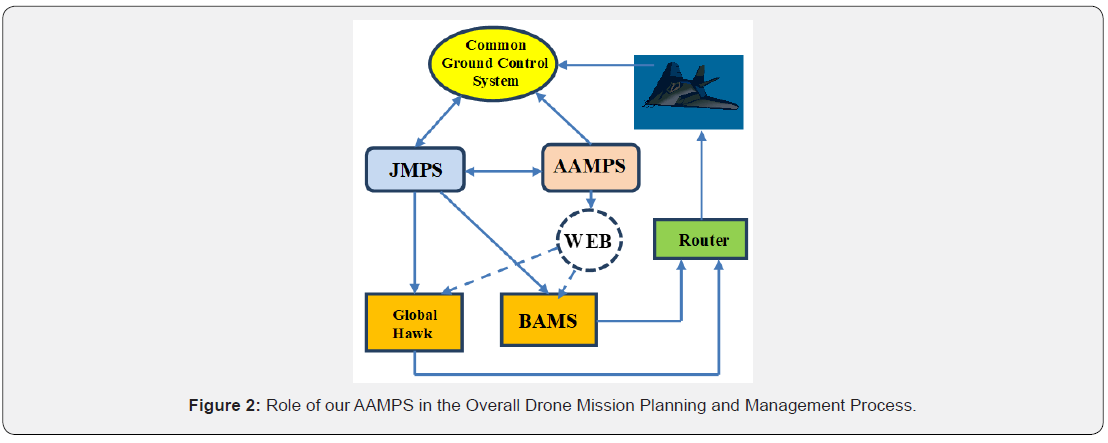
Conclusion
It was argued that RL may not be able to solve contingency
planning for UAVs. Instead, we advocate a practical approach to
solving this problem.
To know more about Robotics & Automation Engineering
Click here: https://juniperpublishers.com/raej/index.php
To know more about Juniper Publishers
Click here: https://juniperpublishers.com/index.php
No comments:
Post a Comment