Neurology & Neurosurgery - Juniper Publishers
Abstract
Background: Electroencephalogram patterns help in evaluating the extent of ischemic brain injury and predicting functional performance.
Aim: To determine a possible correlation between attentional task performance and electroencephalogram waves.
Methods: The cerebral activity of 12 healthy young adults was investigated using an electroencephalogram while they underwent the Trail-Making Test-A and B as attentional tasks.
Results: A significant correlation was observed between a stronger occipital delta power during rest and higher error rates, as well as weaker temporal and central delta power during the Trail-Making Test-B and longer task completion times. Delta waves during both the resting-state and task conditions correlated with task performance, which might be affected by the induced cerebral lobes.
Conclusion: The default mode network might predict attention deficits. Our findings further our understanding of the correlation between the default mode network and attentional task performance.
Keywords: Attentional function; Brain function; Brain injury; Electroencephalography; Neuroimaging
Abbreviations: EEG: Electroencephalogram; DMN: Default Mode Network; TMT: Trail-Making Test
Introduction
Implementation of modular assessments of cognitive capabilities at the individual level is crucial in the realm of personalized care and rehabilitation [1]. Rapid transmission of information across the brain underscores the importance of non-invasive neuroimaging techniques, such as electroencephalogram (EEG) indices. These indices exhibit sensitivity to fluctuations in the human brain, reflecting spontaneous brain activity with excellent temporal resolution [2]. Additionally, EEG signals serve as important neuro-electrophysiological indicators of brain activity [3]. Over the past decade, various new EEG systems have emerged, enabling the recording of brain activity during movement [4]. While a single-dimensional measure, such as pupil size, may be suitable when specific data parameters and goals are well defined, multidimensional signals offer a more viable choice. The EEG gathers information on a variety of functions, including attention, memory, and emotions [5]. Accordingly, the EEG elucidates important effects of brain networks on attentional function from the perspective of brain connections and provides potential physiological biomarkers for predicting attention [6]. Resting-state EEG data can delineate inter-individual variability at rest and its correlation with attentional capacity and autistic behavioral patterns [7]. Different EEG patterns reflect various degrees of ischemic brain injury, with some proving reliable predictors of functional performance [8].
Attention is a fundamental component of all the cognitive and perceptual processes [9]. Similar to other networks in the brain, the human attention system is complex. At any moment, attention can shift between the external and internal stimuli [10]. The literature highlights three primary mechanisms: global, local, and self-attention mechanisms [11]. Attention, as a limited cognitive resource, selectively focuses on discrete aspects of information while ignoring others; it is typically divided into two types: active (top-down or endogenous) and passive (bottom-up or exogenous) [12]. An externally directed attentive state often corresponds to reduced focus on internally oriented mental processes [13]. Sufficiently strong attentive states may impair conscious awareness of both the environment and oneself [14]. Previous studies have revealed the functional roles of alpha (α)-band oscillations [15], which are the most prevalent rhythms in EEG recordings and spread through most cortical regions [15]. However, the role of the alpha wave remains debatable [15]. Elevated parietal α power [16] is associated with a shift from attention to the default mode, which suppresses external stimulus processing [17]. This increase is usually accompanied by an increase in frontal theta (θ) power [16], reflecting cognitive control-task execution, memory function, and error processing [17,18]. Greater θ activity is believed to reflect heightened effort in meeting the increasing cognitive demands of prolonged tasks [16]. Furthermore, heightened power in slow-frequency bands (i.e., θ and α) is associated with lower performance [17]. When the functional state of the brain is suppressed, slow waves with frequencies below 8 Hz occur, namely, δ waves and θ waves [19].
This discourse between power band-associated cognitive performance remains unresolved. To develop novel methods for evaluating attentional function, we must further investigate the fundamental brain mechanisms involved in attentional tasks. Therefore, this study aimed to determine a potential correlation between attentional task performance and EEG waves. By delving into the default mode network (DMN), this study not only unveils insights into attentional deficits but also contributes to our comprehension of the DMN's role.
Methods
Participants
Twelve healthy young adults (seven women and five men; age: 21.3 ± 0.62 years) participated in the present study. All prospective participants were provided with a comprehensive explanation of the study’s safety protocols and were assured that their personal identifying information would remain confidential; thereafter, they provided written informed consent for study participation. Additional informed consent was obtained from all participants whose identifiable information was included in the study. None of the participants had a history of major physical disorders, including neurological illnesses, brain injury, or psychiatric illnesses. This study was approved by the Ethics Committee of Nishikyushu University (approval no. 22EAB19) and conformed to the principles of the Declaration of Helsinki and its later amendments.
Task
The Trail-Making Test (TMT) comprises two distinct parts: Part A requires patients to sequentially connect 25 encircled numbers (through lines) dispersed in a pseudo-random manner on a sheet; In TMT Part B, participants alternate between numbers and letters when connecting different items in an ascending order (i.e., 1, A, 2, B, etc.) [20].
Experimental Setup
Participants were seated in a quiet room on a chair with a backrest and placed their forearms in a relaxed position on a table. They were instructed to perform the TMT without any additional movements, such as head movement, and maintain the same posture throughout the experiment. Additionally, participants were instructed to remain silent throughout the experiment, relax without thinking, and fixate their gaze on a cross displayed on a piece of paper in front of them during both task performance and resting periods.
Experimental Protocol
The experimental protocol comprised two tasks, each preceded by a 1-min rest period. EEG measurements were recorded continuously during the experiment using Polymate Pro MP6100 (Miyuki Giken, Tokyo, Japan). The EEG recorded at the scalp level represents the aggregate currents of the electrical fields generated by neural activity in cortical neural circuits [21]. Prior to electrode placement, the skin was prepared with alcohol, and the electrodes were affixed to an elastic cap using a holder. Based on the international 10-20 EEG placement system, 19 gold-coated active EEG electrodes were placed at specific cortical locations: Fp1 (left frontal pole), Fp2 (right frontal pole), F3 (left frontal), Fz (middle frontal), F4 (right frontal), F7 (left inferior frontal), F8 (right inferior frontal), C3 (left central), Cz (middle central), C4 (right central), P3 (left parietal), Pz (middle parietal), P4 (right parietal), O1 (left occipital), O2 (right occipital), T3 (left mid temporal), T4 (right mid temporal), T5 (left posterior temporal), and T6 (right posterior temporal) (Figure 1).
Data Analysis
The EEG data were sampled at a rate of 1,000 Hz and filtered within the 1-60 Hz range using a bandpass filter. Data containing eye blinks or muscle movement artifacts were excluded. Power spectrum analysis was conducting using the Electro Magnetic Source Estimation Data Editor (Cortech Solutions, Wilmington, NC). Six EEG datasets (delta, theta, alpha, beta, low-gamma, and high-gamma) were used for each electrode. Furthermore, waves lying within the 0-4 Hz, 5-8 Hz, 9-13 Hz, 14-30 Hz, 31-50 Hz, and >50 Hz ranges were categorized as delta, theta, alpha, beta, low-gamma, and high-gamma waves, respectively.
Statistical Analysis
The mean power level was calculated for each resting and task condition. Spearman’s rank correlation was used to examine the correlation between task performance and EEG power levels during rest and TMT tasks. Furthermore, IBM SPSS Statistics version 20.0 (IBM Corp., Armonk, NY, USA) was used for statistical analysis. Statistical significance was set at p <0.05.
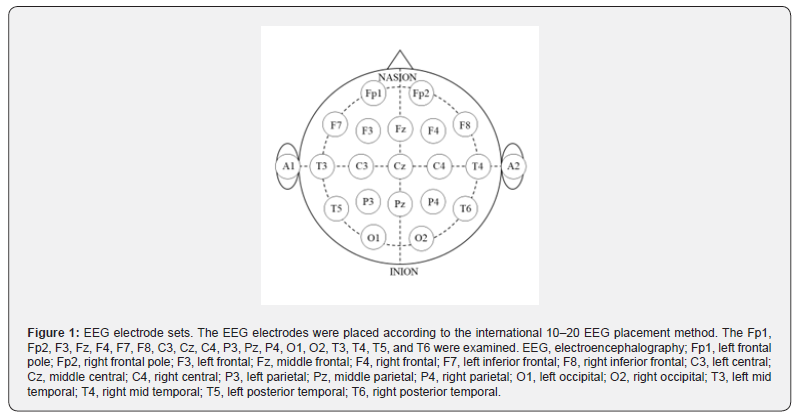
Results
The associations between task performance and EEG power levels during the TMT-A rest and task conditions are summarized in Tables 1 and 2, respectively. Notably, no significant correlations were observed between task performance and EEG power levels for the TMT-A. The correlations between task performance and EEG power levels in the TMT-B rest and task conditions are summarized in Tables 3 and 4. Stronger occipital delta power at rest was significantly correlated with more errors. Additionally, weaker temporal and central delta power during the task were significantly correlated with a longer task time.
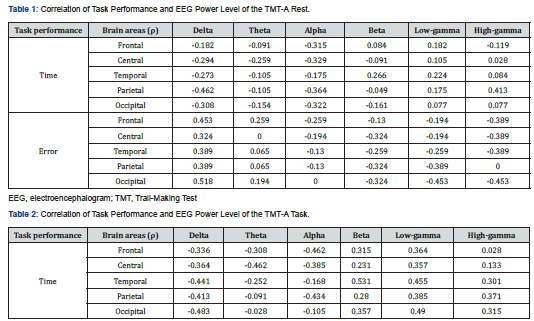
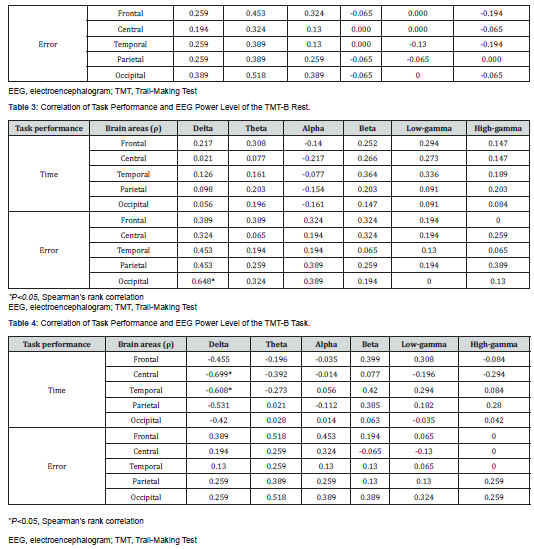
Discussion
Recent studies have suggested that attention operates as a rhythmic process, yet the debate continues regarding whether this rhythmicity is influenced by the phase of the ongoing neural oscillations. Addressing this issue requires the use of behavioral tasks that isolate attention from other cognitive functions (perception/decision-making), and the localized monitoring of neural activity with high spatiotemporal resolution across the brain regions associated with the attentional network.22 In the present study, we investigated the relationship between attentional task performance and EEG frequency using the TMT, which is a simple behavioral task that isolates attention from other cognitive functions. The results showed that a stronger occipital delta power during rest was significantly correlated with more errors. Moreover, weaker temporal and central delta power during TMT-B was significantly correlated with a longer task time. No significant correlations were found between task performance and EEG power levels for TMT-A.
Notably, the modulation of behavioral outcomes through EEG frequencies of 3, 6, and 8 Hz (delta, theta, and alpha bands) throughout the frontal region has been reported in studies focusing on quantifying the phase that predicts the high and low attention states [22]. High amplitudes and percentages of delta waves in the frontal lobe for Fz-F3-F4 demonstrates strong stimulation of the limbic system, strong emotions, flurry thoughts, and confusion [23]. Stress, uncertainty, tension, and the release of adrenaline can contribute to heightened arousal and impact cognitive state [24]. The most dominant patterns of change in patients with attention deficit hyperactivity disorder, schizophrenia, and obsessive-compulsive disorder are power increases across lower frequencies (delta, 1-4 Hz and theta, 4-8 Hz) and decreases across higher frequencies (alpha, 8-13 Hz; beta, 13-30 Hz and gamma, 30-50 Hz) [25]. Impairment of visual attention is associated with differences in EEG features, network activation, and involvement of the right frontal eye field [26]. In contrast, our findings showed that frontal delta power was not correlated with task performance, while stronger occipital delta power during rest was significantly correlated with more errors, and weaker temporal and central delta power during TMT-B was significantly correlated with a longer task time. These results indicate that the influence of delta waves on task performance may be mediated by their interaction with the cerebral lobes, highlighting their role in task specificity. Moreover, these phenomena affect the task performance’s specificity. Furthermore, attentional deficits may be reflected differentially by resting-state and attentional-state EEG data, implying that the DMN may predict attentional deficits.
Additionally, a correlation exists between different EEG frequencies and attentional task performance. A higher theta-beta ratio reflects challenges in attention allocation for a given task [27]. Gamma band activity plays a vital role in many sensory and cognitive processes. Therefore, the peak frequency in the gamma range has received considerable attention [28]. Gamma band activity, combined with frontal midline theta rhythm in the left and right frontal forehead regions, appears to reflect prefrontal function in working memory tasks [29]. Furthermore, lateralized patterns of band phase coupling between the frontal and parietal-occipital regions have been identified during covert visuospatial attention tasks in healthy young adults [30]. While these findings enrich our understanding of the brain mechanisms underlying attention, further exploration into additional EEG frequency considerations and their potential impact on task performance, particularly lateralized patterns, is essential.
This study had some limitations. It focused solely on healthy young adults; therefore, it is unclear whether our results can be generalized to older patients or those with neurological disabilities. Furthermore, the attentional task only utilized the TMT; therefore, whether brain waves during other attentional tasks are comparable to those observed during the TMT remains unclear. Additionally, our study included only a small number of participants. To address these limitations, future studies should include more participants performing tasks under various conditions and investigate brainwave activity across diverse attentional tasks. Despite these limitations, these study findings indicate that stronger occipital delta power during rest is correlated with errors, and weaker temporal and central delta power during tasks is linked to a longer task times, offering insights into the role of brain rhythms in attentional deficits and contributing to our understanding of cognitive functions and neural connections.
Conclusion
Our study identified significant correlations between EEG power levels and attentional task performance. Specifically, stronger occipital delta power during rest was significantly correlated with increased errors, while weaker temporal and central delta power during TMT-B was significantly associated with a longer task time. Delta waves during both the resting-state and task conditions were correlated with task performance, which may also be affected by the induced cerebral lobes. Moreover, our findings suggest the potential predictive value of the DMN for attention deficits. Our findings expand the understanding of the interplay between DMN and attentional task performance, emphasizing the need for further investigations into the intricate relationships between EEG frequencies and attentional task performance.
No comments:
Post a Comment